Your guide to sentiment analysis in customer care
Discover how sentiment analysis can transform your approach to customer care
Add bookmark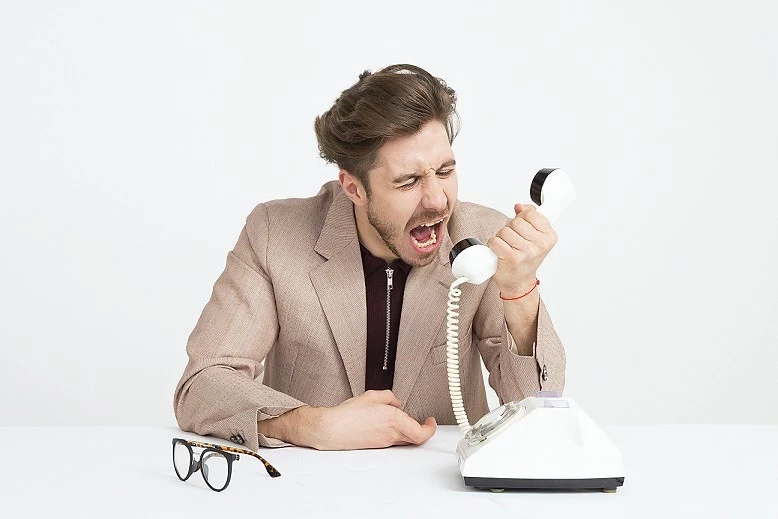
As you will no doubt have heard, data is one of the most valuable commodities for a company, and behind every sentence, statement etc. is a valuable message from your customer.
This article will answer questions, like:
- What is sentiment analysis?
- What are the benefits of sentiment analysis for customer care?
- What are the best practices in sentiment analysis?
What is sentiment analysis?
Sentiment analysis is a way to gauge the mood of your customers through analyzing communications. The communication ranges from direct, such as emails, digital assistant text conversations and in-person speech through to indirect through other media such as social media, Twitter etc.
Benefits of sentiment analysis for customer care
We are all familiar with net promotor scores and other such metrics as a mechanism to try to understand how customers are feeling at the point you ask the question. However, this only works at the point of asking the customer for feedback. Whereas being able to understand how a customer feels at a given point in time in their journey with you is far more valuable, as it enables you to do something in real-time.
There is a range of benefits involved with implementing sentiment analysis, including:
-
Better understanding of your customers
Real-time understanding of how customers feel about your brand allows you to deal with issues at the moment the customer is engaged. If you can deal with a negative issue as a priority, you have an opportunity to change that customer’s perspective about your brand, from negative to positive.
-
Personalized customer engagements
Whether the customer is interacting with an agent or a digital assistant, if the contact is able to modify their behavior to be more empathetic towards the customer, this leads to a more personalized experience and will typically make the person feel better about the engagement.
-
Improve agent skills
Not all agents are perfect all of the time. Identifying when an agent’s actions cause a negative sentiment from the customer, and then using that to train the agent, will improve the agent’s future interactions with customers.
-
Prioritize service requests
Service centres can use sentiment analysis when prioritizing which customers to support as part of the process. Customers with problems that have clearly caused them to feel strongly can be routed to specific agents trained to deal with the specific problems, or those with more experience, and this can be managed as a priority.
-
Track sentiment over time
By being able to track sentiment over time, in near real-time, you can identify quickly what impact changes to processes and products is having. This allows you to be agile in your business and to pivot quickly so, for example, a negative change can be stopped or modified quickly before reputational damage is done.
Best practices
-
Where to start?
The starting point should be your business strategy and goals and from that starting point, you can identify how sentiment analysis can support these.
With a clear strategy, you can identify how this technology can assist in the benefits to customer care as noted above. From this, you can then identify what steps to take to support these goals
-
Good, bad and unsure
One useful trick in sentiment analysis is that if you are classifying the customer response as positive or negative sentiments, then it is advisable to create a third classification called ‘neutral statement’, where the statement is neither positive or negative. For example, stating a known fact cannot be considered as a positive or negative statement. In this way you can ensure that the model created will avoid classifying the statement as false negative or false positive.
-
How to classify sentiment?
It is possible to use a lexicon-based method in sentiment analysis, and the benefit of this is a small set of training data. However, it only works with the simplest written communications.
[inlinead-1]
Using tokenization with lexicon also has an inherent flaw when it comes to sentiment analysis, as one of the initial steps is to convert everything to lowercase, which would miss someone ‘shouting’ in an email, indicated by them using all capital letters.
Machine learning is by far the best approach, with diverse features allowing you to construct a classifier to identify the sentiment within the customer communication. The benefit is this approach can be used for any form of communication. Whether the customer sends emails, uses social media or makes a call and uses their voice, machine learning models can be trained to understand what the customers’ sentiment is.
-
Data
You will no doubt have heard the phrase ‘data is the new oil’ many times by now. Like oil, the more you refine your data the better the quality of the output. Having the right data is challenging so a data strategy should be a key priority when delivering any AI projects. Ensure you have the right data for the type of analysis you want to perform and try to ensure no bias is present in the data. For example, ensure the data used to train the model is a good representation of the communication normally seen for the channel being modelled.
-
Where to listen?
Given the wide variety of possible ways for customers to communicate, both directly and indirectly with a company, it is important to identify the channels you are going to monitor. Identify where you want to initially focus your efforts and start with that channel.
-
Context
Context is critical to understanding why the customer feels the way they do. For example, if the customer is completing a survey, the way they respond is likely to be different than when raising a support ticket. You can also use machine learning to help you understand the context. For example, if the support ticket says, “Room service was excellent but the food was terrible” then you can use machine learning to identify why we have a positive response for one aspect, and a negative for another.
-
Automated action
Being able to analyze the sentiment of the customer in real-time is all well and good, but unless you act on it in real-time there is little point in doing the analysis in the first place. Using sentiment analysis, you can ensure that urgent tickets are escalated to agents as a priority.
-
Holistic solution
It is great to implement sentiment analysis and when combined with automation, digital assistants and other tools such as Intelligent Advisor you can not only streamline your customer care but you can tailor it to provide unique personalized care.
As well as identifying the sentiment of the customer, if you also use machine learning to understand the context then you gain real benefits. For example, if a customer purchased a product for their wife for a wedding anniversary and it arrived damaged they might email the company first and then follow up with starting a complaint via a digital assistant. Automation can identify that this is an emotional contact, that the problem is damaged goods and that the customer has already contacted the company. Now you can have the digital assistant pass the customer directly to a human agent, who is fully briefed on why the customer is calling and that they are angry, and this gives the agent a chance to change the customers’ sentiment by providing a more personalized service.